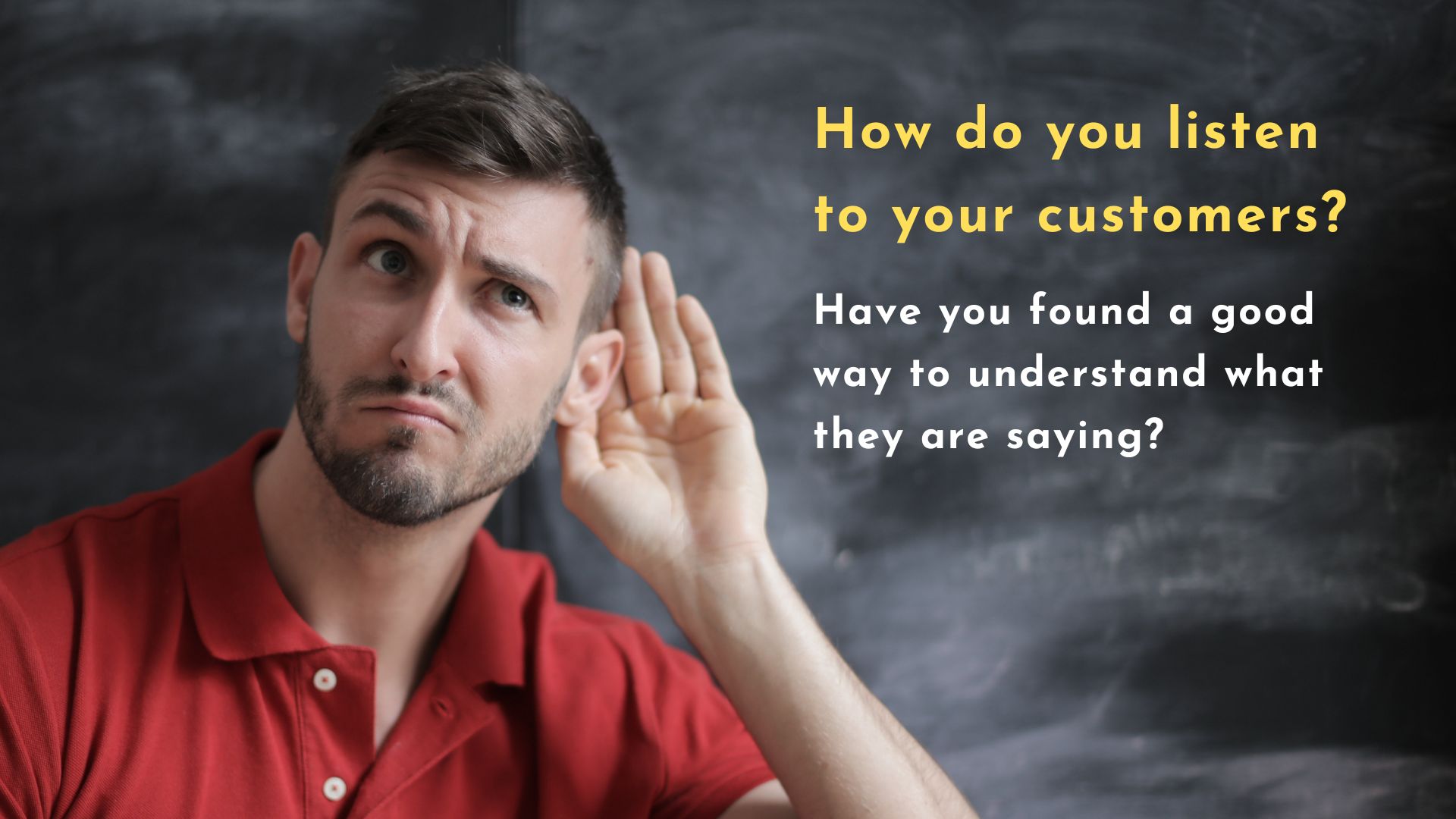
VoC (Voice of the Customer) means what it literally appears, namely what your customers are saying! VoC can include their expectations, their preferences, their feedback, their aversions, and so forth. By truly understanding the voice of your customers, you have better chances to meet their needs and expectations, improve your products and services, and therefore increase your customer loyalty and satisfaction.
Difficulties with Interpretability of numbers solely
With that said, what keeps businesses from substantiating the voice of their customers is the ability to analyze available data. Data consists in various formats, such as numbers, texts, images, and sounds. While numbers are the easiest to be visualized and extracted, it is time-consuming to process texts and difficult to turn them into useful information.
Let’s take N, a fictional subscription-based streaming service company, for example. N has been the biggest streaming service in Sweden for the past years. In order to provide better services, N has been offering various types of content and upgrading its interfaces. However, since the beginning of the year, N has experienced a decrease in the number of subscribers.
When such business wants to find out why the subscribers leave, it typically has quantitative data, such as churn rate, hours watched, types of content watched, what was or wasn’t popular, etc. While it is common to analyze these data, interpretability can be a potential issue. One needs to structure the data in a meaningful way and then interpret them with humanized words. Yet, doing so comes with some uncertainties:
- Different interpretations by different people of the same data;
- The representation of the behavior data to the actual reasons for unsubscribing;
- The complexity of causes leading to unsubscriptions;
- Out-of-date metrics and control systems, and so on.
So, why not look into what the customers have already told you?
Text mining is an eligible way to understand the voice of your customers. Nowadays Artificial Intelligence (AI) is capable of understanding texts contextually and the sentiments in them. You can teach AI models, simply by feeding some historical data, to perform what you intend to. Labeling and structuring the texts in a quantitative way facilitates the scalability of processing data. With the ability to quantify texts and process qualitative reviews, digging deeper into the historical data eliminates the problems of gathering useful information. For example, you could gather the discussions on the forums, customer reviews on the platform, or customer inquiries from your service department, and thus have more data sources to truly understand the reasons behind user behaviors.
Instead of bothering (ex-)customers who want to leave or just left with surveys and interviews, the N team uses AI to data-mine previous customer interactions and reviews. The team analyzes customer feedback and tries to determine what urges them to leave: (1) pricing, (2) the content offered, (3) navigation of the platform, (4) customer service satisfaction, (5) technical and compatible issues, and (6) others.
Finding valuable information from the texts
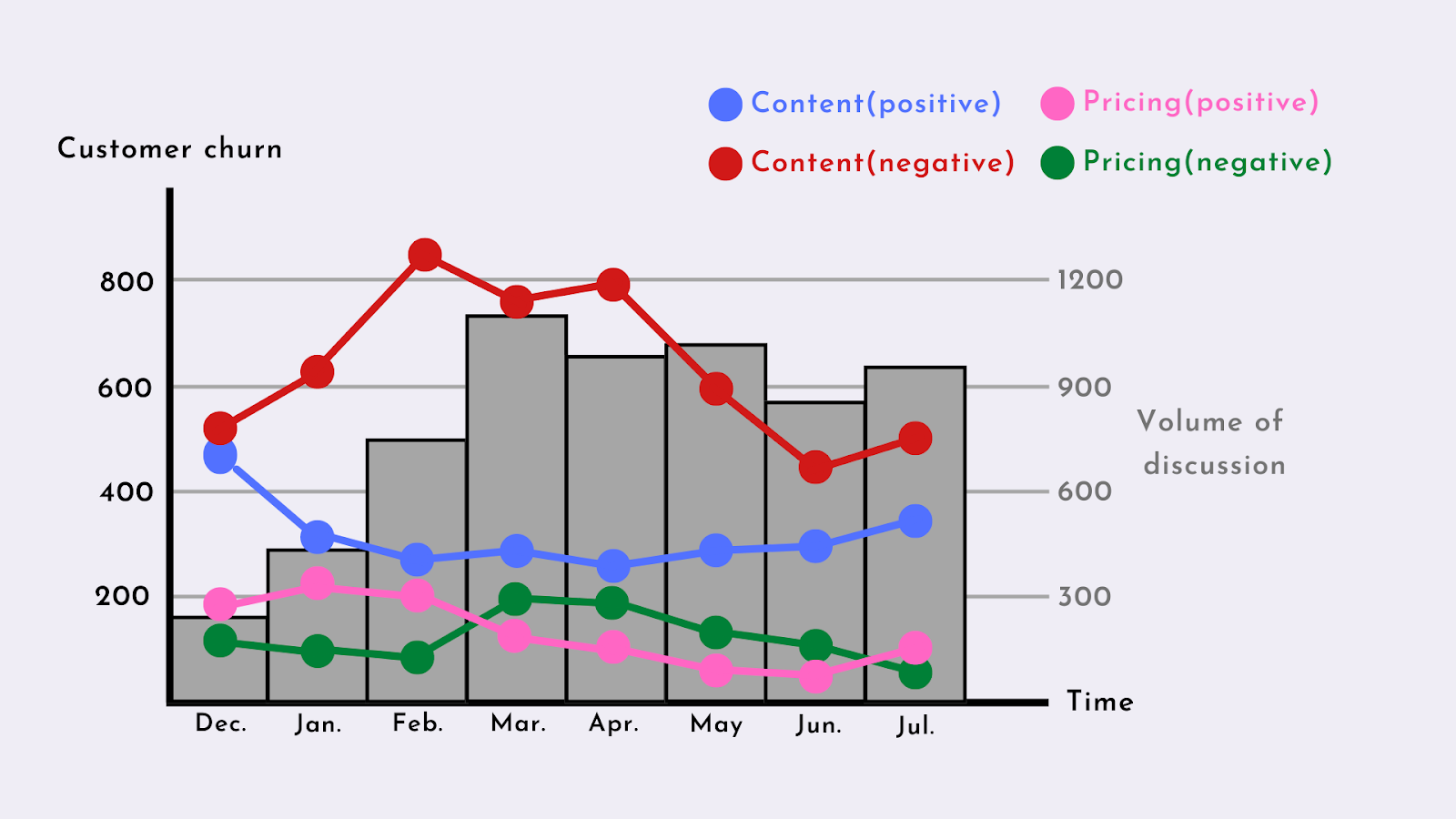
To find out what factors were potentially causing the phenomenon, N processed its user reviews within these time periods and got the results. After quantifying their customer reviews, N team creates a diagram including customer churn and the chosen factors (not fully shown). Clearly, the N is having an issue with customer churn, which reached its peak in March and has stayed high. With designated categories and sentiment analysis, the AI model sorted the texts into different classifications and visualized the review data.
In the diagram, it is clear that for the users, content is more influential to the churn than pricing. From the start, positive and negative discussions were of about the same volume. Yet, in the subsequent months, users were dissatisfied with the content on the streaming platform. Hence, N could trace back to the time and find out what films were launched or taken down at the time. Interestingly, the negative comments about pricing outnumber the positive ones in March, which practically can be compared with competitors’ campaigns or be linked with the content offered in the specific month.
Exploiting the benefits of AI in text analysis
This is just an example to demonstrate how text quantification can create new ways of evaluating your business performance. Apart from the numbers, adding heterogeneous data types provides different approaches to analyzing your user behavior. All in all, understanding the voice of the customers is an absolutely essential task, and including various data types will enable you to understand your customers closer and deeper.
Sounds interesting to you?
Feel free to contact us for more details or sign up for a free trial.